Deep learning tool may help cut emissions caused by air resistance
Aerodynamic drag is a major contributor to global emissions. Here’s how a recent development in deep learning can help reduce it.
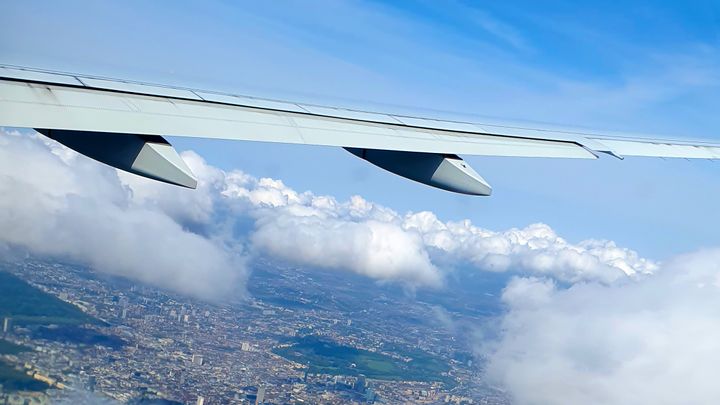
Deep learning tools have revolutionized aerodynamic engineering for planes, cars and ships, enabling these vehicles to be more fuel efficient and structurally refined. A new computational model pushes the science of reproducing airflow further yet, by relying on neural network architecture to generate accurate predictions while saving time, cost and energy.
Researchers at KTH Royal Institute of Technology, along with collaborators in the U.S. and Spain, published the model in Nature Communications, reporting that it delivers a high degree of accuracy in predicting aerodynamic drag with a substantially lower computational cost.
Simple in its design and built using data obtained from more complex simulations, the framework is what’s known as a reduced order model (ROM). As the name implies, they retain the most essential features of more elaborate models while omitting less important details.
“The point is to reduce computational complexity and make simulations or analyses more efficient,” says Ricardo Vinuesa, lead researcher and fluid mechanics associate professor at KTH Royal Institute of Technology. “Design engineering requires is the ability to run many different scenarios at a low computational cost.
“Using this model, we can get quite accurate predictions of many scenarios.”
The use of neural networks is what elevates the model beyond those which engineers have typically used to make predictions out of the chaos of airflow, Vinuesa says
Standard reduced order modeling in fluidics relies on linear computation that—in the most simple terms—produces predictions through adding and scaling values.
By contrast, neural networks are based loosely on the function of the brain.
“Very loosely,” Vinuesa cautions. “That doesn’t mean the model can think for itself, as many people assume.” But what they can do—unlike linear models—is learn and map intricate relationships between input and output data. This is regarded as a valuable capability when attempting the notoriously complex task of predicting and modeling air friction close to the surface of an airplane wing or a train engine.
“We can better predict how the flow around an airplane wing changes over time. If we can predict this better we can control the flow to reduce the drag, and also we can better improve the aerodynamic design of the wing.”
The new model can capture most of the original physics in a flow prediction, 90 percent or more, with relatively little processing complexity, Vinuesa says. By comparison, reaching that level of accuracy is much more complex an operation for state-of-the-art linear models such as proper-orthogonal decomposition (POD) and dynamic-mode decomposition (DMD).
“Linear models basically represent their predictions in a very simple way, by relationships that can be simplified to straight lines and planes,” he says. “But reality is more complicated. That’s why having models that are not based on straight lines, but all kinds of other shapes, enables us to get better predictions.”
Which is important considering that aerodynamic drag is a significant contributor to global emissions.
If used in aerodynamic control, this technology can lead to reductions of the drag of 20, 30 or even 50 percent,” he says. “It can have a significant environmental impact and help determine the type of world warm-up scenario we end up at in the future.
"The environmental and economic consequences are huge.”
Contacts
David CallahanInternational Public Information OfficerKTH Royal Institute of Technology
press@kth.seImages
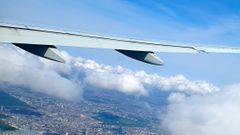
Subscribe to releases from KTH Royal Institute of Technology
Subscribe to all the latest releases from KTH Royal Institute of Technology by registering your e-mail address below. You can unsubscribe at any time.
Latest releases from KTH Royal Institute of Technology
AI helps decode horses' body language for better veterinary care9.4.2025 12:20:55 CEST | Press Release
Researchers are using AI to bridge the communication gap between horse and human. Combining 3D motion capture and machine learning, a new modeling system would equip veterinarians with a powerful visual tool for interpreting equine body language—the key to detecting physical and even behavioral problems.
New Swedish Initiative to Integrate Research and Healthcare4.4.2025 11:37:56 CEST | Press Release
A groundbreaking national initiative, Precision Omics Initiative Sweden (PROMISE), aims to connect research with healthcare and establish Sweden as a world leader in data-driven precision medicine.
For graphene production, a potential green alternative to mining graphite3.3.2025 14:14:40 CET | Press Release
Researchers in Sweden report a green alternative to reduce reliance on mining graphite, the raw source behind the "wonder material" graphene.
AI on aircraft can help prevent stalls and terrifying drops in altitude17.2.2025 15:58:14 CET | Press Release
Artificial intelligence could help prevent terrifying mid-air drops in altitude. In a new study, an international research team successfully tested a machine learning system for preventing trouble with turbulence.
Alternative to studded winter tires reduces airborne particles by 20 percent6.2.2025 14:41:07 CET | Press Release
On icy roads, studded winter tires can save lives – but they pulverize pavement and fill the air with dangerous, inhalable particles. A new Swedish study shows that both road wear and airborne particles could be reduced by as much as 20 percent if studs were made instead with an alternative hard metal.
In our pressroom you can read all our latest releases, find our press contacts, images, documents and other relevant information about us.
Visit our pressroom